
Nonpoint source pollution impairs water quality worldwide, but it is difficult to mitigate because of the varied sources and ways it moves through landscapes. Mitigation relies on best management practices (BMPs), which can be structural (like vegetated buffers or bioreactors) or nonstructural (like minimizing fertilizer use or scheduling irrigation). BMPs are key to the integrity and sustainability of water resources, but they must be effectively designed and implemented.
As part of a multistate research project, scientists at land-grant universities are working together to develop data, models, and monitoring techniques that can be used to detect water quality issues and target, assess, and improve BMPs under various environmental and socioeconomic conditions.
Multistate research makes it possible to address nonpoint source pollution more thoroughly than a single state or institution. Initiated over 20 years ago, this project now consists of scientists from 22 universities with expertise spanning multiple disciplines as well as rural and urban environments from Texas to Vietnam to the Arctic. Long-term cooperation among project members and partners enables sharing of ideas and resources, facilitates efficient research, and drives innovation.
Research-based BMPs help reduce nonpoint source pollution and improve water quality—as well as health of the humans and other species dependent on rural and urban water resources—while ensuring sustainable agricultural production.
Research Highlights (2020-2024)
Over the years, this group has produced findings and tools that have increased understanding of nonpoint source pollution and appropriate BMPs. In 2023 alone, members received 31 research grants, published 80 research papers, developed 10 models, delivered 42 Extension workshops, and mentored 50+ students and scientists. Recently, project members collaborated to develop a special collection for the Journal of Environmental Management, a premier journal which is regularly and widely cited.
Researchers are leveraging artificial intelligence and automated technologies to improve modeling and monitoring. For example, project members developed:
Models that use machine learning to simulate the effects of management practices at a national scale. The U.S. Environmental Protection Agency (EPA) adopted these models as part of the HAWQS national decision support system. Texas A&M
A machine vision and learning system that uses trail camera images and videos to measure stormwater flow from culverts. These techniques have revolutionized culvert monitoring especially in low light. North Carolina State University
The Physics-AI model, which estimates streamflow forecast errors at ungauged locations, improving forecast reliability to about 82% compared to about 21% using traditional methods. Auburn University
A machine learning framework that uses satellite imagery to predict flooding in agricultural landscapes. North Carolina State University, University of Tennessee
BMP-Net, a deep neural network that uses satellite imagery to identify vegetative and structural BMPs worldwide with over 90% accuracy. Texas A&M
A new approach that uses geospatial data, aerial imagery, and AI to detect gully locations on agricultural hillslopes. Kansas State University
A smart web-based tool that makes it easier for users to filter the EPA database and select a total maximum daily load report. These reports can provide useful guidance for developing watershed management plans. Texas A&M
A system that uses drones, game cameras, and GIS to assess groundcover, cattle and wildlife density, and E. coli loading. Oklahoma State University
Sensors and analytics to predict livestock manure excretion and distribution. Sensor data are used to model critical runoff areas and guide an autonomous robot that manages the manure deposits. Virginia Tech
A machine learning-based forecasting system to predict fecal coliform concentrations in shellfish harvesting areas. North Carolina State University
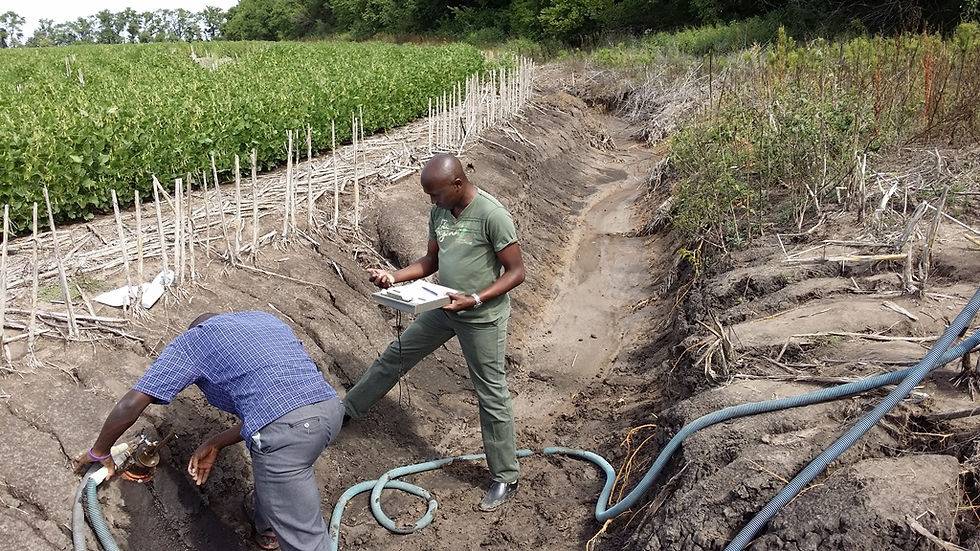
To improve water quality in heartland reservoirs, researchers at Iowa State University, Kansas State University, University of Missouri, and University of Nebraska worked with Extension specialists and farmers to develop gully erosion control practices. In the photo above, scientists test soil erodibility in a gully. Photo by Aleksey Sheshukov, Kansas State University.
Multiple studies evaluated how effectively certain farming practices, including precision farming techniques, mitigate water quality issues. For example, project members:
Monitored and modeled the source, fate, and transport of contaminants in tile-drained landscapes and studied the effect of subsurface drain depth and spacing on water quality and crop yield. Synergistic project with the University of Illinois, Iowa State University, University of Kentucky, and the USDA-ARS in Ohio and Indiana
Identified dairy practices and climate factors that contribute to higher levels of stream pollution and used data to develop a decision support tool that helps target appropriate BMPs in critical watersheds. University of Maryland
Provided more evidence that cover crops can help mitigate runoff and developed a decision support tool for cover crop management. Oklahoma State University; University of Illinois
Used models to identify irrigation scheduling that would result in high corn yields while minimizing nitrate leaching. University of Georgia
Are developing a mechanistic modeling tool (SPOTMOD) that can be used to quantify how much agrochemical runoff is reduced by precision (spot) applications compared to traditional broadcasting methods. This is groundbreaking because there was previously no method for doing so. This tool can be used in regulatory environmental risk assessments in North America and the European Union. University of Florida
Developed the Vegetative Filter Strip Model (VFSMOD) to model pesticide removal by vegetative filter strips. The model is used widely to design optimal filter strips for specific scenarios and to assess the effectiveness of existing strips. It has also been used in environmental risk assessments for the registration of pesticides in North America and the European Union, spearheading a shift away from previous tools that could not account for the variability of agricultural pesticide applications. University of Florida
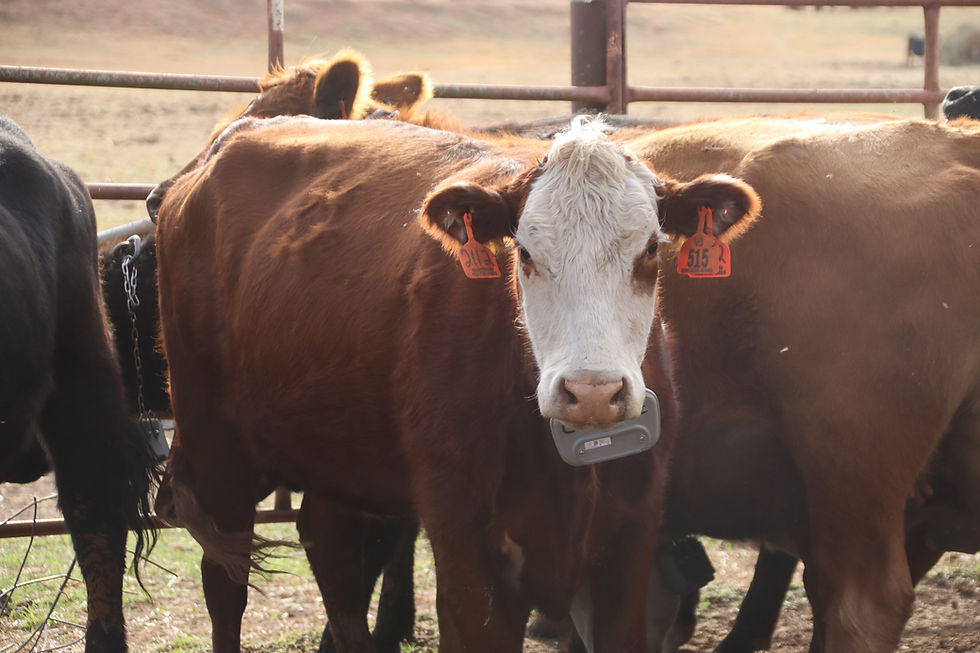
To help protect water quality from cattle grazing, scientists at Oklahoma State University are studying virtual fencing, in which cattle wear GPS collars (shown in the photo above) that provide an auditory cue and electric stimulus when they get too close to programmed boundaries. In recent studies, virtual fencing reduced cattle use of exclusion areas by 78% on average. Based on these findings, the USDA has incorporated virtual fencing in management plans. Photo by Kevin Wagner, Oklahoma State University.
To improve stormwater management, scientists:
Created a stormwater runoff model for suburban areas in southern Rhode Island and evaluated the impact of improved roadside BMPs. University of Rhode Island with the Rhode Island Department of Transportation
Worked with a nonprofit organization to develop and deploy sensors on the Rappahannock River to monitor urban stormwater BMP effectiveness. Virginia Tech
Worked with The Nature Conservancy to quantify the impacts of nature-based stormwater solutions. Kansas State University, Texas A&M
Modeled landscape characteristics that explain pathogen presence in floodwaters with a focus on water quality after Hurricane Florence. North Carolina State University, University of Tennessee

Kansas State University researchers worked with local municipalities to develop and implement stormwater quality programs that incentivize BMP adoption in upstream agricultural areas through partnerships with university research and Extension. This collaboration has already resulted in $6.28 million in upfront cost savings and reduced sediment runoff by 2,672 tons annually. In the photo above, a graduate student takes water samples for laboratory analysis. Photo provided by Kansas State University.
Researchers are monitoring runoff from and developing BMPs for utility-scale solar sites, which are often located in rural areas and require large amounts of cleared land. Virginia Tech
Scientists developed a prediction framework for harmful algal blooms in lakes and reservoirs and evaluated rapid tests for blue-green algae that could be used by Extension agents, producers, and homeowners. Kansas State University
Collaborations with indigenous and international communities have addressed water quality issues worldwide. For example, project members:
Worked with the Prairie Band Potawatomi Nation, area residents, and the Kansas Department of Health and Environment to develop a model and plan for BMP implementation that accounts for specific practices used on the tribal lands. Kansas State University
Organized workshops and worked with the indigenous Sámi communities in Finland and Norway to assess BMPs for water sustainability and reindeer grazing. Kansas State University
Studied how agricultural development and BMP adoption by farmers in Kenya impacts ecological degradation of the dry African savanna. University of Florida
Started working with the Blackfeet Indian Nation in Montana to develop improved prediction of and adaptation to extreme events using artificial intelligence and hydrological forecasting tools that incorporate the community’s traditional ecological knowledge. This groundbreaking work aims to develop tools that can be used by the Blackfeet Nation as well as other underserved communities that have limited monitoring data available to them. University of Florida
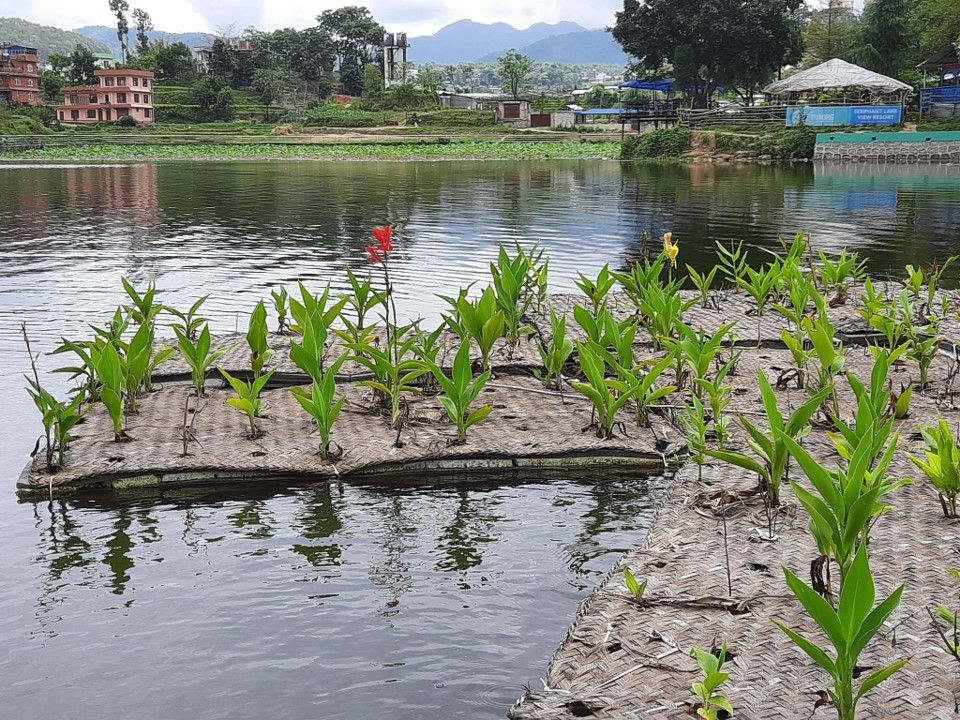
Researchers at the University of Rhode Island collaborated with the Asia Pacific Network and international partners in Nepal, India, Bangladesh, and Japan to study how well floating wetland systems remediate water pollutants. The photo above shows a floating wetland treatment system made out of locally available bamboo mat layered with foam trash materials. Photo by Samayak Prajapati, The Small Earth Nepal.
Project Funding and Participation
This project is supported in part by USDA NIFA through Hatch Multistate Research Fund allocations to participating State Agricultural Experiment Stations at land-grant universities, which include: Auburn University, University of Delaware, University of Florida, Florida Cooperative Extension, University of Georgia, University of Illinois, Iowa State University, Kansas State University, University of Kentucky, University of Maryland, Mississippi State University, University of Missouri, North Carolina State University, Oklahoma State University, Oregon State University, Pennsylvania State University, University of Rhode Island, South Dakota State University, University of Tennessee, Texas A&M University, University of Vermont, Virginia Tech. This is an ongoing project and may include additional participants. Participants may receive funding from additional sources. Project and participant details: https://nimss.org/projects/18705
This project was the Southern Region's nominee for agInnovation's 2024 Excellence in Multistate Research Award for their outstanding collaboration and impacts.
Commentaires